Banks and other financial organisations must digitise their information for quicker storage and retrieval as they process millions of client papers annually. An developing technique called optical character recognition (OCR) makes it possible to extract data from PDFs and photos and convert them to Excel, CSV, and other formats. With the use of OCR, banks can swiftly extract client information from PDF documents, transform it, and make it searchable. This quickens the process of approving loan and new account applications.
What is a bank statement?
A bank statement is a summary of transactions that financial organisations send to account holders each month. It explains how cash flows to and from accounts by displaying specifics in a tabular manner and providing an overview of credits, debits, charges, and settlements. On bank statements, which lenders consult while reviewing applications, time periods and dates for every transaction are printed.
A bank statement is what? In a nutshell, it’s a document that lists, page by page, your financials for a specific month.
What is OCR?
Optical character recognition, or OCR, is the term used to describe the technique used to scan pictures and transform them into readable representations. The characters from papers are extracted, recognised, and processed for electronic conversions in this type of automated data capture method. Organisations digitise and store electronically in databases the characters and letters that OCR software reads. These are then prepared for sharing, access, and viewing by being processed or converted into various file formats. OCR is a tool that banks employ to track customer spending patterns, examine bank statements, and assess customers’ creditworthiness. Accounts payable departments utilise OCR to speed up client onboarding and offboarding procedures, minimise human data entry, and improve corporate operations.
Where is OCR used in the banking sector?
OCR usage in the banking industry may be divided into two categories: usage by banks and usage by clients and other commercial organisations. OCR is used by banks to digitise client data for use in customer onboarding, signature comparison, and other processes.
We’ll see how OCR may be utilised by customers and other non-bank businesses in the remaining sections of the article, particularly to extract information from bank statements.
All owners of bank accounts, whether they are people or businesses, handle bank statements. A bank statement, also known as an account statement, is a document sent to customers by banks that details the transactions made in their accounts over a given time frame. Account holders may manage fees and interest, monitor account balances, and look for identity fraud with the use of OCR-driven data extraction from bank statements. Extracts from bank statements are essential for filing and calculating taxes.
For corporations, data extraction from bank statements acts as a financial record for tax filing procedures as well as a way to track the success of the company. Businesses can utilise information taken from bank statements to evaluate their overall financial health, identify obligations, and figure out deductions.
Customer Onboarding
Address verification during customer onboarding is the most frequent application of bank statements. Customers will be required to submit evidence of address when opening a bank account in order to confirm their residence. In addition to utility bills, housing permits, and other forms of identification, bank statements are a recognised form of evidence of address. The bank statements must be current or older than 90 days in order to be used as evidence of residence. Businesses can analyse bank statements automatically using bank statement OCR to extract information such as account owners’ names, addresses, and issue dates for address confirmation.
Non-banking organisations utilise the information taken from bank statements for tasks including assessing people’s credit scores and verifying their addresses and identities. Account Statements The following fields benefit from OCR.
- Healthcare: For the sake of processing medical bills, insurance claims, and credit, healthcare institutions need bank statements from patients. The central database must be manually updated, which takes time and increases the risk of mistakes. Zonal and AI-enabled OCRs can speed up the process and get rid of mistakes.
- Real estate: To approve a transaction, bank statements from the prospective buyer are frequently required for the acquisition of land and property. For purposes of purchasing, selling, renting, or leasing real estate, bank statements can also serve as evidence of address and identity. Account Statement OCRs can assist real estate agents and brokers in digitising potential customers’ bank statements to speed up the leasing, buying, and renting processes.
- Loans: Bank statements from the applicant are required for the processing and approval of loan applications by banks and other financial organisations. Account Statement These organisations frequently employ OCR to hasten the loan approval procedure.
It’s critical to comprehend a bank statement’s contents in order to comprehend how Bank Statement OCR might be employed, let’s see how in the next section.
The structure and content of bank statements
A bank statement is a summary of all transactions made in a certain account during a specific period of time. It would have to provide the following details.
- Information on the account holder, including account number
- The duration of the assertion
- The account’s starting and closing balances
- income deposits, cash deposits, and other types of deposits.
- Account earnings Interest on the Account Service Charges and Penalties Applied to the Account
- Cash, checks, and other payment methods are used to withdraw money from the account.
- Additionally, it could include facts on the account type and other financial institutions, such as the branch’s name and address.
Depending on the bank, the location and arrangement of the aforementioned information may differ, but generally speaking, an account statement may be physically separated into three parts:
the section that includes information on the account holder, including name, address, phone number, etc.
The section includes account information including the account number, account type (such as savings vs checking), branch information, etc.
The date, description, and dollar values for the credits, debits, interest accrued, and penalties assessed are all part of the transaction.
Steps to automate bank statement processing
Financial companies may now automate data extraction from account statements and handle information more effectively thanks to OCR technology. Automating the processing of bank statements requires carefully scanning and analysing pictures of forms and documents, as well as verifying the data to make sure there are no mistakes or missing numbers.
To automatically read forms and extract data from them, IDcentral’s DeepScan ships with a pre-trained bank statement data extraction API. The platform’s automated bank extract processing involves requires you to upload your scanned bank statements by using the upload feature. Next, you can review and approve your extracted documents after you upload them. If you haven’t previously processed several bank statements, it is a good idea to evaluate fields until the API produces 99% accurate data by double-checking information such as the
- Account holder name
- Account number
- Bank name
- initial balance
- final balance
- Fraud
- Error message
- transaction information
You have the ability to examine and change any instances of inaccurate data that were retrieved from these documents that you identify. You can change and add any missing data if necessary. Once you are satisfied with the data extraction, click “Approve.”
The bank statement information may then be downloaded in Excel, CSV, or JSON file formats.
Why automated bank statement analysis is necessary
In a conventional setup, excel sheets would be manually scanned to do bank statement analysis. Such a procedure is time-consuming and prone to mistakes.
This procedure has been automated by technology suppliers. Now, bank statement analyzers can scan PDFs that clients upload and make creditworthiness inferences. To cut down on the time and resources required for analysis and fraud checks, they are packaged with screen scraping techniques like optical character recognition (OCR) and others.
Analysing bank statements as part of a digital lending process
To underwrite a consumer, digital lending uses a variety of data points, both standard and nontraditional. Traditional data like pay, turnover, average balance, cash flow, existing debt and liabilities, investment, and insurance may be retrieved via bank statement analysis.
The core of credit underwriting is comprised of these findings. By adding AI/ML capabilities and performing a multi-factor analysis of payment histories, credit appetite, and transaction behaviours, digital bank statement analysis enables the improvement of traditional underwriting. To learn more about the customer’s purpose and capacity to repay a loan, these insights can be combined with other, device-sourced data.
IDcentral’s DeepScan Document Extraction Solution
The following advantages are available when converting bank statements into digital forms using IDcentral’s DeepScan Document Extraction API solution:
- Flexibility: DeepScans’ deep learning algorithms are capable of handling a wide range of common data constraints, including handwritten text, multiple languages, low-resolution images, images with new or cursive fonts and varying sizes, images with shadowy text, tilted text, random unstructured text, image noise, blurred images, and many more.
- Customizability: Training DeepScans’ OCR models using proprietary/custom data enables the company to better satisfy particular client needs. Depending on the bank and the kind of account, bank statements come in different formats.
- Organisations with many types of accounts in several banks would benefit greatly from the ability to train OCR models to recognise varied forms.
- Adaptability to changes: DeepScans’ OCR models have the ability to quickly retrain current models with fresh data in order to accommodate unanticipated changes.
- Thus, it is simple to manage new data-gathering needs or change bank document formats.
- Identifying tables For the digitalization of bank statements, automatic recognition of tables with structured row-column information is especially helpful.
- Tables may be exported from DeepScan in a variety of formats, including CSV, Excel, and JSON.
- Post-processing is not necessary since important data are automatically extracted and sorted into intelligently designed fields, which reduces the need for human post-processing.
- works with languages other than English or several. For multinational operators who operate across international borders, this functionality is crucial.
- Utilisation simplicity, batch processing of numerous documents, and smooth 2-way interface with various accounting applications.
Conclusion
The DeepScan Solution from IDcentral has the potential to boost corporate productivity and make automated data collection from financial statements simpler. Intelligent document processing is made feasible by machine learning techniques. OCR APIs may also do sophisticated analysis while performing automated data entry and extraction. Simply put, IDcentral’s DeepScan pre-trained API becomes better at processing your account statements the more bank statements you provide.
Try IDcentral’s AI enabled Document Extraction solution – DeepScanRequest a demo
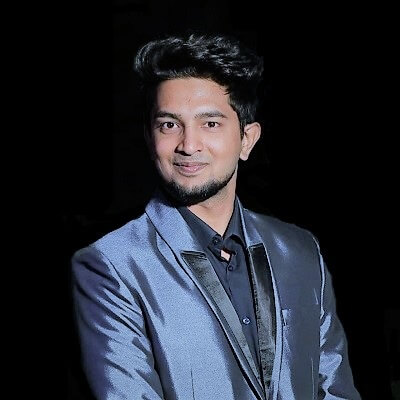
Sumanth Kumar is a Marketing Associate at IDcentral (A Subex Company). With hands-on experience with all of IDcentral’s KYC and Onboarding Technology, he loves to create indispensable digital content about the trends in User Onboarding across multiple industries.